Quality and Risk Awareness for Data Products and Services
Ensuring Excellence & Mitigating Hazards in the Data-Driven Era
Why This Training?
In the era of data-driven decision-making, maintaining quality and understanding potential risks in data products and services is non-negotiable. Poor data quality and unforeseen risks can lead to flawed decision-making, compliance issues, and even financial losses. Equip yourself with the tools, techniques, and insights to ensure your data products are of impeccable quality and understand how to identify, assess, and mitigate risks associated with them.
Duration: 6 Hours (online / virtual live session)
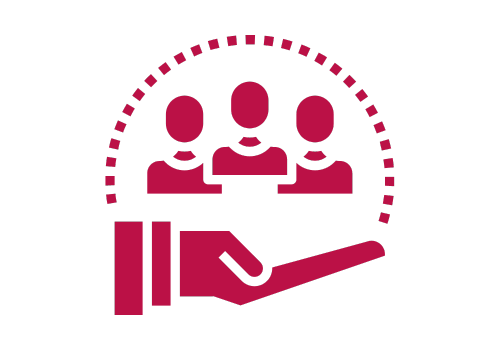
Who Should Attend?
Data Professionals: Data Scientists, Data Analysts, Data Engineers
Project Managers & Team Leads: Those overseeing data-centric projects
Project Managers & Team Leads: Those overseeing data-centric projects
See more
Data Stewards and Data Governance Officers: Professionals responsible for data quality and management
Business Analysts: Those leveraging data for business insights
IT & Tech Teams: Professionals developing or managing data platforms and services
Decision Makers: CTOs, CIOs, and other leaders relying on data for strategic decisions
Business Analysts: Those leveraging data for business insights
IT & Tech Teams: Professionals developing or managing data platforms and services
Decision Makers: CTOs, CIOs, and other leaders relying on data for strategic decisions
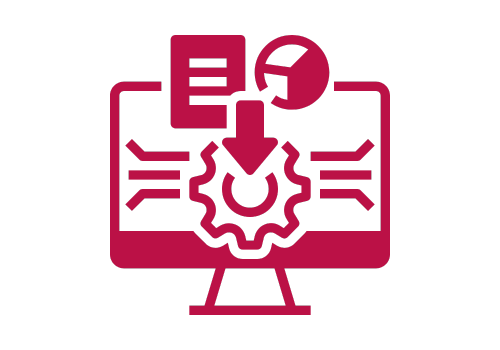
Course Highlights
Dive deep into the principles of Data Quality Management.
Learn to identify, assess, and categorize data-related risks.
Master data quality tools and techniques for modern data platforms.
Learn to identify, assess, and categorize data-related risks.
Master data quality tools and techniques for modern data platforms.
See more
Gain insights into risk mitigation, including anonymization, backup, and disaster recovery strategies.
Understand challenges and strategies in ensuring quality and mitigating risks in Big Data and AI environments.
Participate in real-world case studies and group discussions to contextualize learning.
Build an organizational culture that prioritizes data quality and risk awareness.
Understand challenges and strategies in ensuring quality and mitigating risks in Big Data and AI environments.
Participate in real-world case studies and group discussions to contextualize learning.
Build an organizational culture that prioritizes data quality and risk awareness.
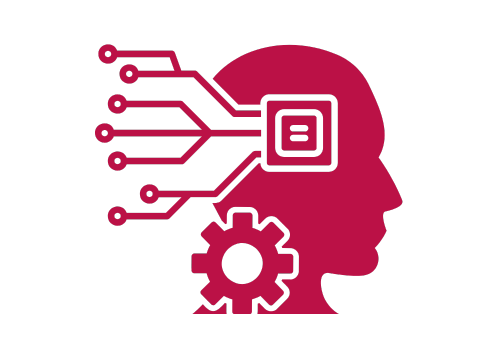
Pre-requisites
Basic understanding of data management principles.
Familiarity with common data platforms and tools (e.g., databases, data warehouses).
No prior knowledge of risk management is required, but a general awareness of data security principles will be advantageous.
Familiarity with common data platforms and tools (e.g., databases, data warehouses).
No prior knowledge of risk management is required, but a general awareness of data security principles will be advantageous.
Training Materials Needed by Participants
A laptop or tablet with internet access for interactive sessions and hands-on exercises.
Any data management or analytics tool you commonly use (for practical sessions).
Notepad and pen for taking notes.
Pre-training reading material (will be shared prior to the training session).
Write your awesome label here.
Training Content
Quality and Risk Awareness for Data Products and Services
Session 1: Introduction to Quality and Risk in Data Products and Services
Understanding Quality in Data
- Definitions and importance of data quality for businesses and operations
- Data accuracy, completeness, relevance, timeliness, and consistency
Principles of Data Quality Management
- Aligning data quality with business objectives
- Continuous monitoring and improvement of data sources
- Importance of data lineage and traceability
- Metadata and documentation
Introduction to Risk in Data Services
- Understanding data-related risks: breaches, misinformation, inaccuracy
- The balance between data accessibility and data security
Risk Identification and Assessment for Data Products
- Tools for data risk identification: Data audits, anomaly detection, and profiling
- Risk categorization: Privacy risks, security risks, compliance risks, and business decision risks
- Evaluating data risks using the risk matrix: Understanding likelihood and impact
Session 2: Delving Deeper into Quality and Risk Management for Data Products
Quality Tools and Techniques for Data
- Data cleansing and validation techniques
- Data quality frameworks: DAMA, TDWI
- The role of ETL (Extract, Transform, Load) processes in ensuring quality
Risk Mitigation and Management in Data Services
- Data anonymization and pseudonymization
- Data backup, archiving, and disaster recovery strategies
- Data breach response plans
Quality and Risk in the Era of Big Data and AI
- Challenges posed by unstructured data and real-time data streams
- Risks of bias and ethics in AI-driven data services
- Ensuring quality in Machine Learning models and outputs
Creating a Culture of Quality and Risk Awareness for Data Teams
- Role of Data Stewards and Data Governance teams
- Training and continuous education tailored for data professionals
- Promoting a transparent data culture with open communication
Case Studies & Group Discussion
- Real-world examples of successful and failed data projects
- Analyzing data challenges and how organizations navigated them
Conclusion & Actionable Takeaways
- Best practices for ensuring quality and managing risks in data projects
- Further resources for mastering specialized data topics.
WOMEN AI ACADEMY
Women AI Academy is a gender-equality and technology driven learning & development organization
Site Terms & Info
ETHOS AI Training & Consulting GmbH
Weihenstephanerstr.1281673
Munich-Germany
We are driven by the vision of making AI both ethical and accessible to everyone
Copyright © 2024 Brought to you by Ethos ai AI Training & Consultancy GmbH
Ali Hessami is currently the Director of R&D and Innovation at Vega Systems, London, UK. He has an extensive track record in systems assurance and safety, security, sustainability, knowledge assessment/management methodologies. He has a background in the design and development of advanced control systems for business and safety-critical industrial applications.
Hessami represents the UK on the European Committee for Electrotechnical Standardization (CENELEC) & International Electrotechnical Commission (IEC) – safety systems, hardware & software standards committees. He was appointed by CENELEC as convener of several Working Groups for review of EN50128 Safety-Critical Software Standard and update and restructuring of the software, hardware, and system safety standards in CENELEC.
Ali is also a member of Cyber Security Standardisation SGA16, SG24, and WG26 Groups and started and chairs the IEEE Special Interest Group in Humanitarian Technologies and the Systems Council Chapters in the UK and Ireland Section. In 2017 Ali joined the IEEE Standards Association (SA), initially as a committee member for the new landmark IEEE 7000 standard focused on “Addressing Ethical Concerns in System Design.” He was subsequently appointed as the Technical Editor and later the Chair of P7000 working group. In November 2018, he was appointed as the VC and Process Architect of the IEEE’s global Ethics Certification Programme for Autonomous & Intelligent Systems (ECPAIS).
Trish advises and trains organisations internationally on Responsible AI (AI/data ethics, policy, governance), and Corporate Digital Responsibility.
Patricia has 20 years’ experience as a lawyer in data, technology and regulatory/government affairs and is a registered Solicitor in England and Wales, and the Republic of Ireland. She has authored and edited several works on law and regulation, policy, ethics, and AI.
She is an expert advisor on the Ethics Committee to the UK’s Digital Catapult Machine Intelligence Garage working with AI startups, is a Maestro (a title only given to 3 people in the world) and expert advisor “Maestro” on the IEEE’s CertifAIEd (previously known as ECPAIS) ethical certification panel, sits on IEEE’s P7003 (algorithmic bias)/P2247.4 (adaptive instructional systems)/P7010.1 (AI and ESG/UN SDGS) standards programmes, is a ForHumanity Fellow working on Independent Audit of AI Systems, is Chair of the Society for Computers and Law, and is a non-exec director on the Board of iTechlaw and on the Board of Women Leading in AI. Until 2021, Patricia was on the RSA’s online harms advisory panel, whose work contributed to the UK’s Online Safety Bill.
Trish is also a linguist and speaks fluently English, French, and German.
In 2021, Patricia was listed on the 100 Brilliant Women in AI Ethics™ and named on Computer Weekly’s longlist as one of the Most Influential Women in UK Technology in 2021.